Artificial intelligence (AI) is a hot topic and increasingly important in product development. But how can this technology be effectively integrated into development projects? Together with our client Audi, we put it to the test and examined the potential and challenges of a machine learning (ML) application – a subset of AI – in a real project. For this purpose, we chose a crash management system (CMS). It is both simple enough to achieve a meaningful result and complicated enough to adequately test the general applicability of the ML method.
Expertise as the Key
ML can only be effectively utilized to the extent the underlying data foundation allows. Therefore, the expertise of the professionals involved plays a critical role. For example, design engineers enter their knowledge of manufacturing and spatial constraints, usable materials, and dependencies into the CAD model. Calculating engineers share their expertise on the simulation process, while data scientists assist with sampling and evaluation.
The creation of thousands of design and corresponding simulation models, as required for the use of Machine Learning (ML), presents a tremendous challenge without automation. The FCM CAT.CAE-Bridge, a specially developed plug-in for CATIA, enables seamless automation across all process steps. Additionally, it embeds all simulation-relevant information (material, properties, solver, and more) directly into the CAD model. The fully automatic translation into a simulation file is done via tools such as ANSA or Hypermesh.
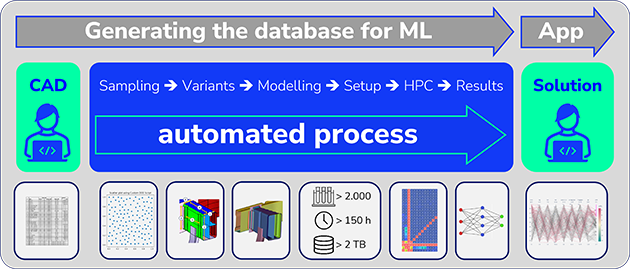
Precise Linking of Parameters and Results
Our approach ensures that the relationship between the CAD model and the simulation model is fully preserved. The automated calculation and evaluation of the models based on specific results create an excellent data foundation for the ML process. The vectors of input parameters with corresponding result values form the basis for the ML approach—clear and comprehensive.
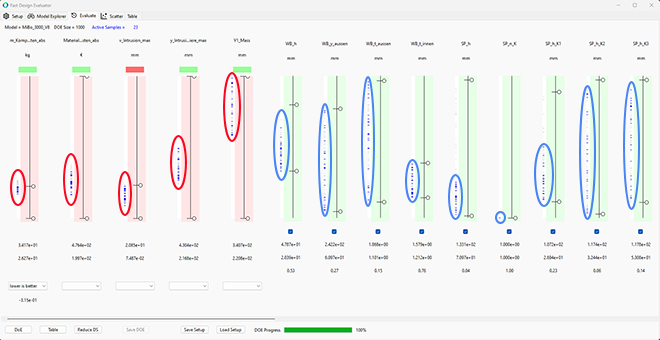
With the trained models and their known accuracy, parameter variations can be quickly tested, and the impact on behavior can be derived—literally within minutes. Once the optimal parameters are identified, they are automatically transferred to the CAD model and the design process can continue.
Conclusion
Our project demonstrated that ML is a valid method for design engineering. The combination of parametric CAD models, simulation, and machine learning provides an efficient approach to making design decisions quickly and accurately. The prerequisite for this is a robust database and the collaboration of the relevant experts on the model. The successful results from the Audi project demonstrate the potential of our data-based approach for product development.