Today, companies face multiple challenges in launching increasingly complex products to the market faster. In particular, the lack of specialized knowledge available from simulation experts in the field of computer-aided engineering (CAE) often slows down product development. Easy-to-use CAE applications can remedy this situation and significantly improve the way products are developed and optimized.
Isolated expertise as a bottleneck in product development
However, daily practice reveals that answering supposedly simple questions, such as the effects of a material change on the deformation behavior of a component or the functional consequences of minor, production-related changes to the component geometry, via simulation, still demands significant organizational effort.
Complex issues require the exchange of numerous pieces of information between the involved process partners. Examples of this include providing current CAD statuses on the part of the design department or feeding back existing test results into the simulation. In addition, relevant decision deadlines and available simulation capacities must be considered. The execution and evaluation of the simulation usually demands specialized expertise, often isolated in expert groups and only available to a limited extent.
Providing access to expert knowledge throughout the organization
Therefore, the goal should be to break down barriers to using simulation technologies, making them accessible to a broad user group – regardless of their technical expertise. The way to achieve this can be termed as “technical democratization of simulation”. It involves integrating existing technical know-how into intuitive CAE applications and making them available to all users company-wide through a CAE business layer.
Three steps to the CAE business layer:
- Analysis
The initial step involves a thorough inventory of the existing CAE processes within the company. This helps to identify the most important processes based on their relevance to the application and to decide which ones are suitable for the development of a CAE application according to the cost-benefit principle. - Standardization
The next step is the standardization of the identified CAE processes which needs the expertise of the calculation engineers. The requirements for the input factors of the CAE process, such as necessary parameters and data, as well as the desired output from the CAE process, are clearly defined. Since simulation processes are typically a complex interplay of different software tools, particular attention is paid to error handling in case potential issues arise during the ongoing process. - Automation
Subsequently, the CAE application is developed and implemented in the company. Deployment on a software platform available throughout the company, which also hosts the data required and generated for the process, ensures comprehensive traceability
Successively, a CAE business layer is created which unites the CAE applications.
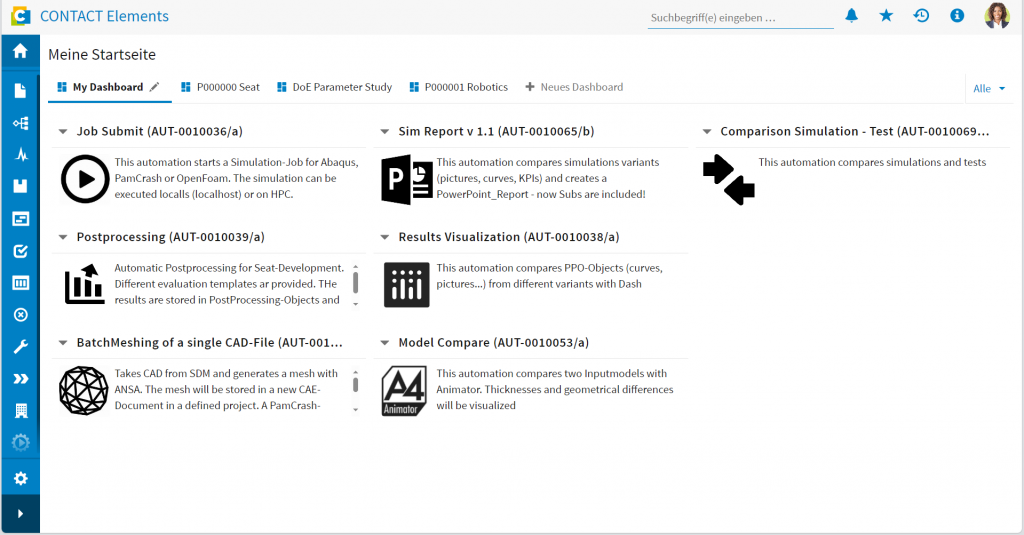
CAE apps dashboard in CONTACT Elements (© CONTACT Software)
Concerns and opportunities
Broad access to simulation technologies does not mean everyone becomes an expert but users are guided through complex processes. An integrated error-handling system reacts to incorrect inputs or deviations in the expected data. Experience shows that expert skills and simulation expertise are not devalued. On the contrary, experienced engineers with a wealth of practical experience and methodological know-how remain indispensable. Through general usage, they can focus on more challenging tasks, accompany decision-making processes, or concentrate on the advancement of simulation methods.
Conclusion: User-friendly CAE applications combine efficiency and innovation
The company-wide provision of user-friendly CAE applications marks an opportunity to establish simulation methods even earlier and more consistently in product development. More users are involved in the process, utilization of resources improves, innovations can be advanced more efficiently and enhanced products will be brought to market in less time. At the same time, it allows simulation experts to focus on more demanding tasks.